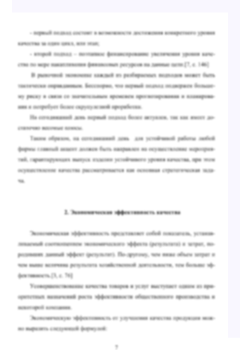
Аналитика больших данных в индустрии путешествий: кейс аффилиатного маркетингового канала компании Aviasales
Цель настоящего исследования заключалась в создании визуализации состояния глобального рынка аффилиатного (партнерского) маркетинга на рынке путешествий в 2020-2021 годах с целью оценки положения компании Aviasales относительно конкурентов. С использованием инструментов аналитики больших данных и машинного обучения в Python, была проведена обработка, моделирование и интеграция в единый массив данных, предоставленных компанией Aviasales. Финальный массив данных был использован для создания аналитического инструмента (дэшборда) в Power BI, с целью оценить характеристики и конкурентную среду мирового аффилиатного маркетинга в индустрии путешествий. Этот аналитический инструмент позволил создать управленческие рекомендации для компании Aviasales касательно развития их аффилиатной программы.
Introduction 8
Chapter 1. Business Understanding 12
1.1. Description of affiliate marketing channel 12
1.2. Company and industry information 13
1.3. Research problems and goal 15
1.3.1. Gap analysis 15
1.3.2. Research goal & tasks 15 1.4. Assessment of situation 16 1.4.1. Research assumptions & limitations 16 1.4.2. Overview of available IT resources 17 1.4.3. Data problems 18 1.5. Project specifications 19 1.5.1. Data mining outputs expectations 19 1.5.2. Research objectives 19 1.6. Project plan 23 1.6.1. Project timeline 23 1.6.2. Project team 23 1.6.3. Research framework 24 1.6.4. Methodological framework 24
Chapter 2. Data Mining & BI Tool Development Process 25
2.1. Data description and exploration 25 2.1.1. General overview 25 2.1.2. Data specification 26 2.1.3. Primary data description 27 2.1.4. Supplementary data description 30 2.1.5. Data problems (detailed description) 32
2.2. Data construction of the direct advertisers’ datasets 34
2.3. Model building for travel advertisers’ classification 35 2.3.1. Selecting modeling technique 36
2.3.2. Model design 39 2.3.3. Model building 41 2.3.4. Model assessment 42 2.4. Data construction for network advertisers’ datasets 43 2.4.1. Domain search by deep link analysis 43 2.4.2. Domain search by advertiser ID (key) 44 2.4.3. Travel class retrieval from affiliate network links 45
2.5. Data construction of the travel verticals classification 46
2.6. Data integration of the final dataset 58 6
2.6.1. Standardization of datasets 59
2.6.2. Join of datasets 60 2.7. BI dashboard preparation 61 2.7.1. Defining affiliate market characteristics 61 2.7.2. BI tool requirements 62 2.7.3. BI tool framework selection 63 2.7.4. Dashboard design 65 2.7.5. Dashboard content 68 2.8. Conclusions on data mining & BI tool development process 77
Chapter 3. Evaluation and Deployment 77
3.1. Validation of results 78
3.2. Affiliate market analysis 80
3.3. Competitive analysis 86
3.3.1. Competitive quadrants 86
3.3.2. Aviasales competition 89 3.3.3. Aviasales affiliate ecosystem 93
3.4. Business recommendations 97
Conclusion 98 References 100 Appendix 103
More and more travelers are searching for airplane tickets, hotels or car rentals using the Internet creating a huge demand for the services and products offered by online travel companies. Oxford Economics estimated the global tourism industry to grow 3.9% annually over the next decade, while a study conducted by Deloitte pointed out that digital technologies would open new opportunities for emerging travel companies and redefine customers’ experience. (Travelpayouts Blog, 2017)
Competition among online travel companies is getting tougher as more players are entering the market offering their customers better prices and wider selection of available products/services. Tough competition requires travel tech companies to look for various ways of marketing their services and generating additional conversion. After accounting for the COVID-19 pandemic impact on the travel industry, the competition can be expected to increase even more.
One of the channels that travel brands actively employ for promotion is affiliate marketing. Affiliate marketing is a type of online marketing whereby a firm (an advertiser or a merchant) makes an agreement with another firm/individual (a publisher or an affiliate) to feature a link from its websites on affiliates sites.
The goals of affiliate marketing are to promote the product and generate sales through additional distribution channels, as well as to increase web traffic to the advertiser’s website in exchange for commission. Commission payable to affiliates is determined by the individual advertiser rewards model, and is usually based on a certain percentage of sale generated by the affiliate. (Dwivedi, et al., 2017) Affiliate programs are employed by a vast majority of travel tech companies, allowing them to generate additional traffic and revenue at comparatively low cost in a highly competitive and price-sensitive environment of the travel industry. That’s why travel companies should thoroughly analyze the potential gains of launching their affiliate programs and understand the current competitive landscape within affiliate marketing channel.
Despite the fact that affiliate marketing existed for some time, there are very few academic papers that were written in the last years regarding analysis and research of the affiliate marketing channel. The first academic literature review was conducted only in 2017 and most of the available studies relied on limited data. (Dwivedi, et al., 2017) Literature review conducted by Dwiwedi, et al. in 2017 showed that most of the sources used by researchers were either purely conceptual or included limited case studies, interviews and secondary data. Few academic studies and lack of quality data clearly shows that the features of an affiliate marketing channel in the travel industry are poorly researched and understood.
8
This research provides an expanded overview on the state of an affiliate marketing channel in the travel industry compared to other studies. It relies on the big data analysis of major characteristics of the affiliate marketing channel in the travel industry and accounts for the effect of the pandemic.
The main research goal of this study was to visualize the state of the global travel affiliate market 2020-2021 in order to assess the position of Aviasales relative to its competitors using data collected by Aviasales team.
Because the data was unstructured, disintegrated and lacked certain descriptive parameters, in order to achieve the research goal, it was decided to initiate the project to tackle the following tasks:
1. Combine all datasets into single data file for further analysis by employing data cleaning, data construction and data integration procedures (data mining stage)
2. Choose characteristics to analyze and appropriate visualizations; prepare a BI dashboard based on combined data file and with the chosen visualizations (visualizations development stage)
3. Analyze broad affiliate marketing conditions and Aviasales competitive position to derive managerial implications (data analytics stage)
In pursuing those tasks, various tools of data mining and data analytics were employed, including web cloud storage (Amazon Web Services), JupyterHub environment with Python libraries (NLTK, Scikit-learn, PySpark) and business intelligence software (Microsoft Power BI).
This research has theoretical as well as practical value. In terms of theoretical value, it is the first quantitative assessment of an affiliate marketing channel in the travel industry in academia. As for the practical value, this research formed the basis for the analysis of the competitive environment in travel affiliate marketing that can be used by the management of the Aviasales company for better decision-making and understanding of the channel.
Advertisers and affiliates in affiliate marketing were the objects in this study, while general processes, parameters and characteristics of the advertisers and affiliates were the subject.
Overall approach for structuring work related to data mining was defined by the Cross-Industry Standard Process for Data Mining (CRISP-DM) methodology. In addition, several natural language processing and machine learning methods were used for modeling purposes, including text tokenization and lemmatization, term-frequency inverse-document-frequency and k-means clustering algorithms.
9
This research relied on primary data on affiliate links and advertisers provided by Aviasales company. Theoretical background regarding affiliate marketing, data mining, natural language processing and machine learning methods were supplied by the academic papers, articles and studies. Frameworks and methodology for analyzing an affiliate marketing channel were developed in collaboration with Aviasales industry experts. Finally, this research relied on technical documentation for Python programming language NLP, ML and Big Data libraries, such as NLTK, Scikit-learn and PySpark.
Structurally, the research is divided into three chapters. The first chapter provides an overview of the business-related background. It defines research the goal, tasks, objectives, limitations and resources for all stages of the project – data mining, visualization development and data analytics. The second chapter provides a detailed overview of the data mining and visualization development work completed. The last chapter is focused on utilizing the created BI dashboard to describe global travel affiliate market characteristics and develop managerial recommendations for Aviasales based on the competition analysis.
Data mining work for the research is organized according to the CRISP-DM methodology principles outlined in the work of Chapman, et. al in 2000 (see Table 1 below).
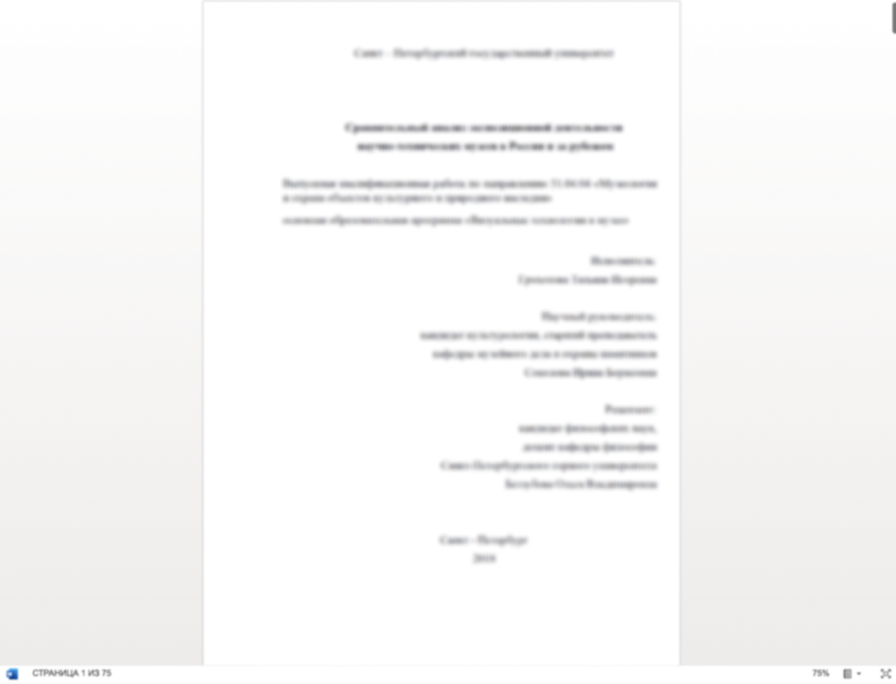
Хочешь уникальную работу?
Больше 3 000 экспертов уже готовы начать работу над твоим проектом!