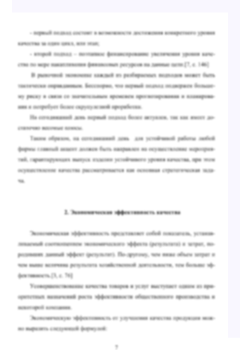
Предпосылки краха платформ на многосторонних рынках
Основной целью работы было определение факторов для предсказания краха платформ. В результате проведения анализа были выявлены проблемы в применении традиционных методов оценки бизнеса в оценке платформ; была построена предикативная модель, позволяющая спрогнозировать риск краха платформы, основываясь на исторических данных из открытых источников.
INTRODUCTION ……………………………………………………………………………………………5
CHAPTER 1. Platform overview and specifics ……………………………………………………7 1.1. Platform definition and typology……………………………………………………………………….. 7 1.2. Network effects and multi-sided markets………………………………………………………….. 14 1.3. Comparison between platforms and conventional value chains…………………………… 17 Chapter 1 findings ……………………………………………………………………………………………….. 20
CHAPTER 2. Platform valuation…………………………………………………………………….21 2.1. Platform strategies …………………………………………………………………………………………. 21 2.2. Assessment of platforms potential……………………………………………………………………. 23 2.3. Issues of platform valuation…………………………………………………………………………….. 24 2.4. Reasons for platforms failure ………………………………………………………………………….. 31 Chapter 2 findings ……………………………………………………………………………………………….. 37
CHAPTER 3. Platforms failure premises determination ……………………………………38 3.1. Methodologyanddatasamples……………………………………………………………………….38 3.2. Key concepts and metrics ……………………………………………………………………………….. 44 3.3. Model and outcomes of quantitative analysis…………………………………………………….. 46 3.4. Analysis of results ………………………………………………………………………………………….. 50 Chapter 3 findings ……………………………………………………………………………………………….. 54
CONCLUSION ……………………………………………………………………………………………… 55 REFERENCE LIST ……………………………………………………………………………………….57 APPENDIX …………………………………………………………………………………………………… 62
The world’s most valuable public companies and its first trillion-dollar businesses are built on digital platforms that bring together two or more market actors and grow through network effects. The top-ranked companies by market capitalization are Apple, Microsoft, Alphabet (Google’s parent company), and Amazon. Facebook, Alibaba, and Tencent are not far behind. As of January 2020, these seven companies represented more than $6.3 trillion in market value, and all of them are platform businesses.1
Platforms are also remarkably popular among entrepreneurs and investors in private ventures. When a 2017 list of more than 200 unicorns was examined (startups with valuations of $1 billion or more), estimation showed that 60% to 70% were platform businesses. At the time, these included companies such as Ant Financial (an affiliate of Alibaba), Uber, Didi Chuxing, Xiaomi, and Airbnb.2
The thorough research of the studies on platforms and their valuation has shown that the majority of the researchers focus on factors that guarantee platforms’ efficiency and the reasons to stick to this business model. However, the previous research on the evaluation of platforms is limited and there is no discussion of any hints or premises of platforms failures. The majority of such papers outline typical managerial mistakes that lead to failures of the platforms. In regard of this research gap, this paper is aiming to study the factors that can be used in platform evaluation and, thus, can reveal premises of default.
The problem is that platforms fail at an alarming rate. It seems vital for managers and investors to identify the sources of companies’ failures and, therefore, avoid the obvious mistakes. Traditional way to assess company’s value as of an investment target – are financial ratios derived from its financial statements, rating agencies reports and accounting information. Thus, promising, growing and successful companies differ from those that have high chances to default or to lose their share of the market by combination of financial ratios and metrics.
Platforms significantly differ from other types of organization: the platform business model encompasses specific features of financials, operations, contracts, communication, stakeholders, and thus, specific risks and opportunities for the business.
This paper’s aim and main goal is to determine factors that predict the failures of platform organizations.
1 Based on public stock market valuations on Jan. 22, 2020: Apple, $1.4 trillion; Microsoft, $1.3 trillion; Alphabet/Google $1 trillion; Amazon, $937 billion; Facebook, $633 billion; Alibaba, $602 billion; Tencent, $483
billion.
2 “The Crunchbase Unicorn Leaderboard,” TechCrunch, accessed July 2017, https://techcrunch.com.
5
Objectives:
1) to study and discuss the features of platform organizations that distinguish them
from conventional firms
2) to study specifics of multi-sided market;
3) to discover and discuss the valuation issues concerning platforms;
4) to analyze reasons of companies’ failure that have been discussed in previous
researches;
5) to define factors that can be premises of platforms failure;
6) to develop and test a predicative model with some of those metrics embedded as
factors of failure prediction for platform organizations.
Subject of the research – premises of companies’ failures. Object of the research –
platforms organizations that operate in multi-sided markets
The main assumption of this work is that organizations with platform business models
differ significantly from conventional pipeline companies. Therefore, the valuation of such companies is different and should be based on other factors than financial metrics and market values. There is a hypothesis that a set of non-financial factors embedded into a mdoel might be a tool to predict platform’s failure. Thus, the expected results are 1) to reveal the insignificance of traditional financial approaches used to evaluate the companies’ performance and probability of collapse in case of platforms, 2) to determine the factors that make the analysis and evaluation of platforms significant and help predict default of company of that type.
The first chapter of this paper will be devoted to nature of platforms, features of multi- sided markets and distinctions between platform and pipeline types of organizations. The second chapter will contain the discussion of issue in platforms’ valuation and observation of previous researches focused on platforms’ failures. The third chapter is aiming to determine a combination of factors that can be regarded as premises of platforms’ failures via applying the multiple discriminant analysis (MDA). The managerial implication approached in this thesis is that the final list of factors embedded in the predicative model can be used as premises for companies’ failure.
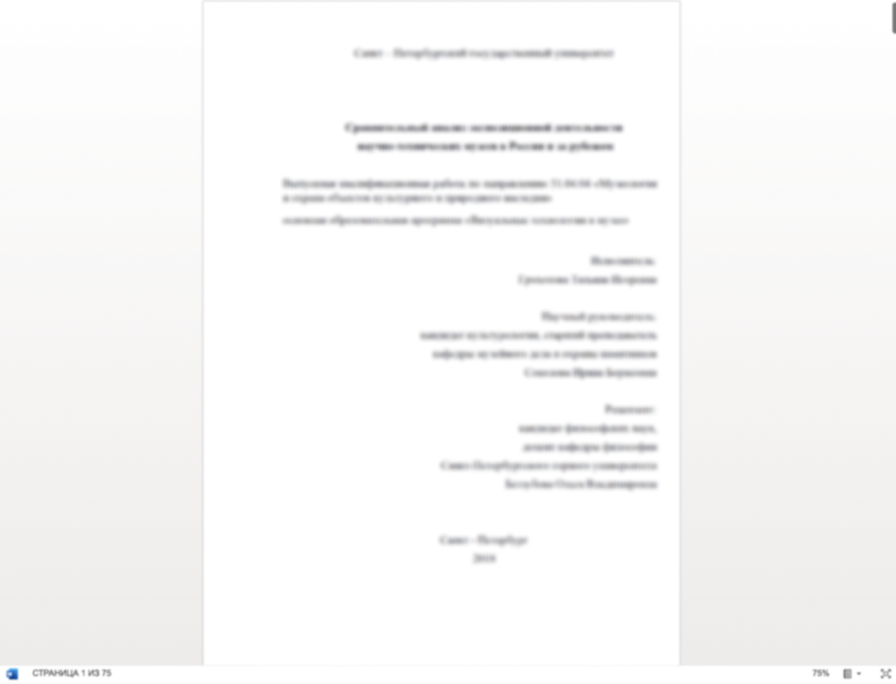
Хочешь уникальную работу?
Больше 3 000 экспертов уже готовы начать работу над твоим проектом!