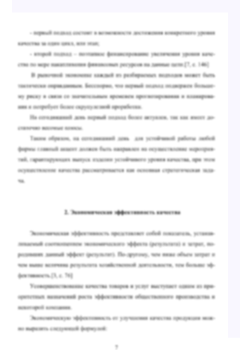
Создание модели оттока в компании и формирование процессов по его снижению
Создание модели оттока клиентов на сегодняшний день является одной из наиболее актуальных задач в области дата саенс для телекоммуникационной отрасли в России. Как и компании во многих других отраслях по всему миру, мобильные операторы в России столкнулись с сокращением потребительского спроса и ужесточающейся конкуренцией в 2020 году. Своевременное выявление пользователей, которые собираются сменить мобильного оператора, позволяет компании заранее начать деятельность, направленную на удержание таких клиентов, и, таким образом, сохранить выручку. Целью данного проекта является создание модели оттока клиентов для компании TELE2 и предложение процессов по его снижению. В результате проекта была создана модель машинного обучения, которая позволит компании выявлять наиболее уязвимых клиентов, которые собираются отказаться от услуг оператора. Более того, в работе было предложено несколько экономических последствий, которые можно использовать для оценки затрат на кампании по удержанию и потенциального увеличения прибыли. Наконец, была создана блок-схема процесса сокращения оттока, в которой выделены ключевые области улучшения для компании, а затем были предложены конкретные шаги по улучшению процесса.
TABLE OF CONTENTS 5 INTRODUCTION 6
CHAPTER 1. CUSTOMER CHURN ROLE IN TELECOMMUNICATION MARKET AND TOOLS TO HANDLE IT 8
1.1. Definition of churn 8
1.2. Telecommunications market overview 10
1.3. Literature review 14 1.3.1. Predictive modelling approaches and results 14 1.3.2. Business implication of the models 19
CHAPTER 2. CHURN PREDICTION MODELLING AND IMPLICATION OF RESULTS 22
2.1. Metric of quality 22 2.2. Exploratory data analysis 25 2.3. Feature engineering & Holdout dataset 29 2.4. Dimensionality Reduction 29 2.5. Handling sample imbalance & Data generation 30
2.6. Models used and results 32 2.6.1. K-nearest neighbours 32 2.6.2. Logistic Regression 34 2.6.3. Random Forest 36 2.6.4. XGBoost 38 2.6.5. ANN 39 2.6.6. Models results discussion 42
2.7. Managerial implications 44
CONCLUSION 49
REFERENCES 51
APPENDICES 55
Appendix 1. Code for Exploratory data analysis 55 Appendix 2. Code for Feature engineering & Holdout dataset 58 Appendix 3. Code for Dimensionality Reduction 59 Appendix 4. Code for Handling sample imbalance & Data generation 60 Appendix 5. Code for training models 62 Appendix 6. ANN training history 69
The world is developing at very high speed and competition is arising everywhere, especially in technological spheres, where knowledge spillovers are very fast. Telecommunication services is one of the industries with fierce competition and where the bargaining power of customers is high due to their low switching costs between mobile operators. Russian market, which is of our interest, is one of the most technologically advanced and has vast potential for development.
To increase revenue and profit, mobile operators need to increase their user base. There are two ways to achieve this. Companies can attract new users or they can reduce the outflow of existing users. It is true for both the international and Russian telecom market that retaining one user is cheaper than attracting one new user. Several studies have demonstrated that attraction of a new customer is usually around six times more costly than retaining an existing one. (Colgate & Danaher, 2000) However, user retention measures are also quite expensive and the company cannot carry out user retention measures for all customers.
Apart from the aforementioned facts, the relevance of the paper is justified by the volume of the market. The Russian mobile telecom market is saturated and approaches 200%, meaning that almost every person has 2 SIM-cards. Therefore, as the time goes, it is getting harder to attract new customers and these new customers are often the most price-sensitive ones from other companies.
Creating customer churn models is one of the most pressing data science challenges for the industry. Identifying users who are going to change their mobile operator in time allows the company to start activities aimed at retaining such customers in advance. This paper focuses on creation of a churn prediction model and giving recommendations for churn reduction using the data provided by Russian mobile operator TELE2.
TELE2 is one of the most popular telecom operators in Russia. It was established in 2003 and now operates in 65 regions of Russian Federation, providing services in high-speed internet connection of 3G/4G standard. The main track of a company’s development is creation of products with the best available price-quality ratio. It has significant success among clients and has become the fastest growing operator by average year revenue from services. To sum up, the
6
current approach allowed the company to not only attract the price-sensitive customer segment, but also capture the most growing segment of digital clients, who use the data (internet access).
There are two important points in building customer retention processes in the company. Firstly, retention of the customer does not happen instantly, which means that company needs to start retention measures before the user decides to change mobile operator. The second important point is that some users bring more profit to the company than others, which means TELE2 needs to differentiate customer retention measures and spend more resources on retention of customers, which are important for the company. The object of research in this paper is the actual churn of the customers in telecom mobile operators. The subjects of this paper are the created customer churn prediction models.
The remainder of the paper proceeds as follows. Firstly, churn definition will be stated according to both the industry and TELE2 data. Then, most popular international and Russian practices for churn prediction will be reviewed. After that, most common problems with modelling will be regarded and solutions will be discussed. In the empirical part, preliminary data analysis will be performed, results of different models will be presented and compared. Final section gives conclusions, ideas for further research development and discusses research limitations.
The goal of the project is to create a churn model for the company and suggest processes for churn reduction. Considering the project objectives, they are the following:
1. Define churn.
2. Choose type of the model (regression, binary classification, multiclass classification)
3. Determine how much time is required for customer retention measures and based on this determine the prediction horizon for the model.
4. Choose a quality metric.
5. Build a model on historical data.
6. Suggest an experiment design for testing models on real users.
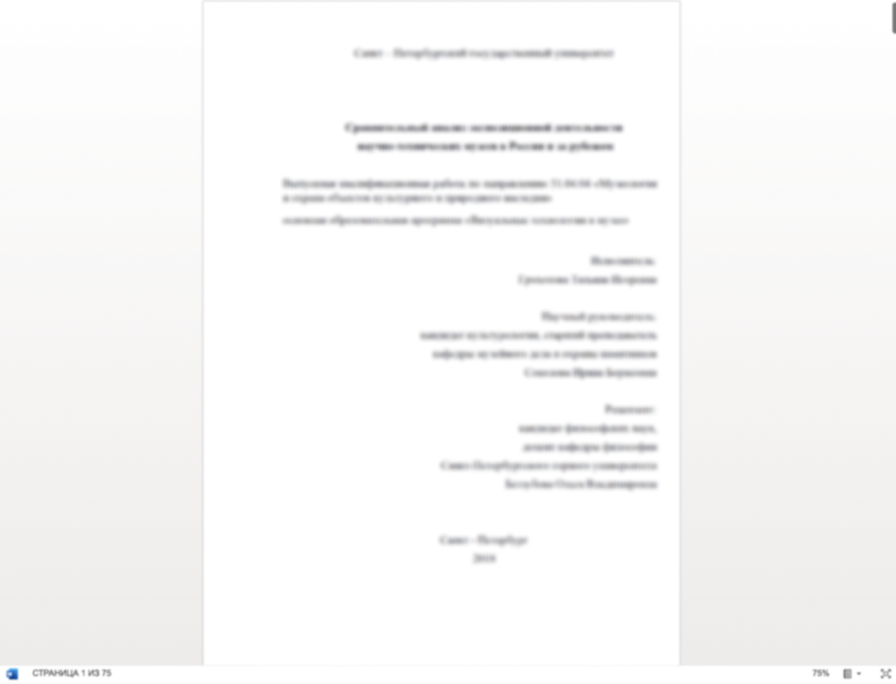
Хочешь уникальную работу?
Больше 3 000 экспертов уже готовы начать работу над твоим проектом!