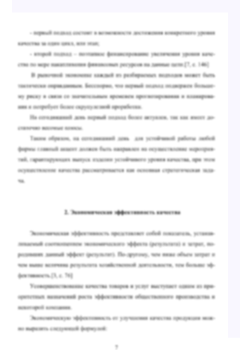
Исследовательский анализ данных и оценка эффективностей университетов с использованием DEA и SFA моделирования
Исследование посвящено разработке рейтинга эффективности российских университетов, входящих в мировые рейтинги университетов, с помощью математических подходов оценки эффективности – data envelopment analysis и stochastic frontier analysis. В исследование проведён полный цикл разработки рейтинга – от сбора данных на веб-сайтах с помощью веб-скрепинга до применения моделей. Важными составляющими проведённого анализа также является доказание несостоятельности мировых рейтингов университетов с помощью статистических методов, исследовательский анализ данных и использование метода главных компонент для снижения размерности данных. Для решения указанных задач реализованы программы на языках программирования Python и R.
Introduction 3 Problem Statement 4 Literature review 5
1 Chapter 1. Benchmarking 8
1.1 TheoryofProduction………………….. 8 1.2 Efficiencymeasurementapproaches …………… 9 1.3 DataEnvelopmentAnalysis ………………. 12 1.4 StochasticFrontierAnalysis ………………. 14 1.5 PrincipalComponentAnalysis……………… 18
2 Chapter 2. Data Processing 21
2.1 Datacollection …………………….. 21 2.2 Rankingsconsistency………………….. 23 2.3 Inputsandoutputs …………………… 25 2.4 ExploratoryDataAnalysis……………….. 30
3 Chapter 3. Modeling 35
3.1 DEAranking ……………………… 35 3.2 SFAranking………………………. 36 3.3 Results…………………………. 38 3.4 Furtherdevelopment ………………….. 39
Conclusion 40
Bibliography 41
Appendix 45
Appendix1.Programmingcode……………….. 45
Efficiency measurement of company’s activity plays a vital role in its further development. Managers can assess information how certain depart- ment works and compare it with other departments and branches of the company. This information is crucial, when it is necessary to allocate money and resources inside the company, open new branch or close the existing one.
It is remarkable that not only commercial organizations try to improve their efficiency. Many nonprofit organizations such as public universities, charity funds, society institutions and their activity can be also estimated by numerical approaches.
World university rankings are the most common way to compare uni- versities in different countries across all continents. They are used for about 30 years since they were first developed and they become more and more important guideline for numerous universities. Although these rankings are comprehensive and trustworthy, there are many issues associated with them: small amount of attributes, controversial attributes, different weights of at- tributes. Also, there rankings do not use solid mathematical model, but just a few formulas to aggregate attributes. The purpose of the research is to provide a mathematically consistent analysis to compare universities using well-known intelligible benchmarking approaches such as Data Envelopment Analysis (DEA) and Stochastic Frontier Analysis (SFA).
The purpose of the research was the development university rankings for Russian universities presented in all world university ranking using modern benchmarking approaches – data envelopment analysis and stochastic frontier analysis.
Required preliminary steps were done: overview of literature, data col- lection, exploratory data analysis, dimensionality reduction of inputs and outputs in order to use SFA modeling.
Classical and modern scientific publications were analysed, several ef- ficiency measurement approaches were introduced with the most important one – Farrell efficiency.
An important step is data collection which was done using two web scrapers written in Python. They gathered data and organized it for further analysis.
Exploratory data analysis helps to understand data and choose the most important variables for further analysis. Also, it shows some interesting facts about universities, such that there are some universities which have dormitory square more than educational and research square.
Principal component analysis was used because there was a problem when the number of observations was less than the number of variables. As some inputs and outputs are highly correlated, it was easy to aggregate them into principal components.
The results of DEA and SFA modeling show that all Russian universi- ties are almost equally efficiency. While DEA ranking assigned equally rank 1 to all universities, SFA showed the distinction in the fourth decimal place
[1] Benito, M., Gil, P. & Romera, R. Funding, is it key for standing out in the university rankings?. Scientometrics 121, 771–792 (2019).
[2] Bougnol, M., Dula ́, J.H. Technical pitfalls in university rankings. High Educ 69, 859–866 (2015) doi:10.1007/s10734-014-9809-y
[3] Bougnol, M., Dula ́, J.H. Validating DEA as a ranking tool: An application of DEA to assess performance in higher education. Ann Oper Res 145, 339–365 (2006) doi:10.1007/s10479-006-0039-2
[4] Brock, A. Budgeting models and university efficiency: A Ghanaian case study. High Educ 32, 113–127 (1996).
[5] Buela-Casal, G., Guti ́errez-Mart ́ınez, O., Bermu ́dez-Sa ́nchez, M.P. et al. Comparative study of international academic rankings of universities. Sci- entometrics 71, 349–365 (2007) doi:10.1007s11192-007-1653-8
[6] C ̧akır, M.P., Acartu ̈rk, C., Ala ̧sehir, O. et al. A comparative analysis of global and national university ranking systems. Scientometrics 103, 813–848 (2015).
[7] Chen, K., Liao, P. A comparative study on world university rankings: a bibliometric survey. Scientometrics 92, 89–103 (2012).
[8] Daraio, C. and Bonaccorsi, A. (2017), Beyond university rankings? Gen- erating new indicators on universities by linking data in open platforms. Journal of the Association for Information Science and Technology, 68: 508-529. doi:10.1002/asi.23679
[9] De Filippo, D., Casani, F., Garc ́ıa-Zorita, C. et al. Visibility in interna- tional rankings. Strategies for enhancing the competitiveness of Spanish universities. Scientometrics 93, 949–966 (2012).
[10] Elken, M., Hovdhaugen, E. & Stensaker, B. Global rankings in the Nordic region: challenging the identity of research-intensive universities?. High Educ 72, 781–795 (2016).
41
[11] M. J. Farrell Journal of the Royal Statistical Society. Series A (General) Vol. 120, No. 3 (1957), pp. 253-290.
[12] Go ́mez, I., Bordons, M., Fern ́andez, M.T. et al. Structure and research performance of Spanish universities. Scientometrics 79, 131–146 (2009).
[13] Johnes, J. University rankings: What do they really show?. Scientomet- rics 115, 585–606 (2018).
[14] Kao, C., Pao, H. An evaluation of research performance in man- agement of 168 Taiwan universities. Scientometrics 78, 261 (2009) doi:10.1007/s11192-007-1906-6
[15] Khalil Jahangiri email ; Ali Rezazadeh1; Mohsen Poorebadollahan2
[16] Lovell CAK (1993). “Production Frontiers and Productive Efficiency.” in Fried HO and SS Schmidt (eds.) the Measurement of Productive Effi- ciency: Techniques and Applications, Oxford U.K.: 3-67.
[17] Moed, H.F. A critical comparative analysis of five world university rank- ings. Scientometrics 110, 967–990 (2017).
[18] Piro, F.N., Sivertsen, G. How can differences in international uni- versity rankings be explained?. Scientometrics 109, 2263–2278 (2016) doi:10.1007/s11192-016-2056-5.
[19] van Raan, A.F.J., van Leeuwen, T.N. & Visser, M.S. Severe lan- guage effect in university rankings: particularly Germany and France are wronged in citation-based rankings. Scientometrics 88, 495–498 (2011) doi:10.1007/s11192-011-0382-1.
[20] Pietrucha, J. Country-specific determinants of world university rankings. Scientometrics 114, 1129–1139 (2018) doi:10.1007/s11192-017-2634-1
[21] Robinson-Garc ́ıa, N., Torres-Salinas, D., Delgado Lo ́pez-C ́ozar, E. et al. An insight into the importance of national university rankings in an inter- national context: the case of the I-UGR rankings of Spanish universities. Scientometrics 101, 1309–1324 (2014) doi:10.1007/s11192-014-1263-1.
42
[22] Saf ́on, V. What do global university rankings really measure? The search for the X factor and the X entity. Scientometrics 97, 223–244 (2013).
[23] Salas-Velasco, M. The technical efficiency performance of the higher ed- ucation systems based on data envelopment analysis with an illustration for the Spanish case. Educ Res Policy.
[24] Schwekendiek, D. Recent changes in World University Rankings: an explorative study of Korea and Germany. Asia Eur J 13, 361–377 (2015).
[25] Shehatta, I., Mahmood, K. Correlation among top 100 universities in the major six global rankings: policy implications. Scientometrics 109, 1231–1254 (2016) doi:10.1007/s11192-016-2065-4
[26] Yudkevich, M., Altbach, P.G. & Rumbley, L.E. Global university rank- ings: The “Olympic Games” of higher education?. Prospects 45, 411–419 (2015) doi:10.1007/s11125-015-9365-y
[27] Xueqian Zhang, Yalin Qian & Qiangqiang Zhao, Performance Evalua- tion of Scientific Research in China’s First-class Universities from the Per- spective of Multi-agent Based on Malmquist index, SE-DEA and SFA Re- spectively. 2nd International Conference on Economy, Management and Entrepreneurship (ICOEME 2019), doi:https://doi.org/10.2991/icoeme- 19.2019.64.
[28] Timothy J. Coelli, D.S. Prasada Rao, Christopher J. O’Donnell, and George E. Battese. An Introduction to Efficiency and Productivity Anal- ysis. Springer Science+Business Media, Inc., 2005.
[29] Peter Bogetoft, Lars Otto. Benchmarking with DEA, SFA and R. Springer Science+Business Media, LLC., 2011.
[30] QS World University Rankings – Top Universities,
https://www.topuniversities.com/university-rankings
[31] World University Rankings — Times Higher Education (THE),
https://www.timeshighereducation.com/world-university-rankings
43
[32] ARWU World University Rankings 2019 — Academic Ranking of World Universities
http://www.shanghairanking.com/
[33] CWUR — Center for World University Rankings https://cwur.org/
[34] Monitoring — Information and analytical materials based on the results monitoring the quality of training http://indicators.miccedu.ru/monitoring/?m=spo
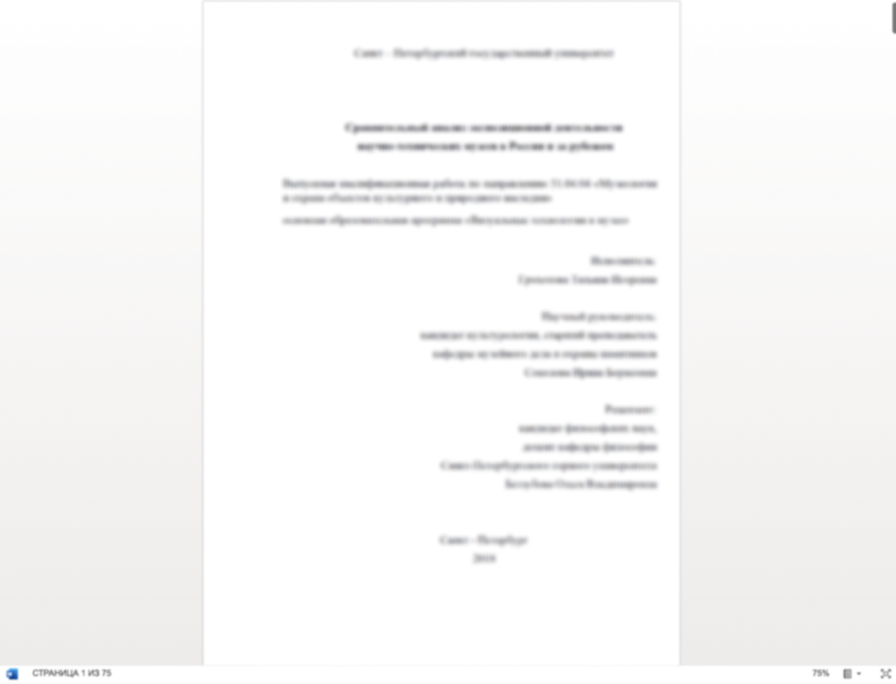
Последние выполненные заказы
Хочешь уникальную работу?
Больше 3 000 экспертов уже готовы начать работу над твоим проектом!